In the recent Digital Pacemaker podcast between Uffective’s CEO Mark van der Pas, and Uli Irnich and Markus Kuckertz of Vodafone (you can listen to it in German here), one of the topics they discussed was watermelon projects.
When senior leadership evaluates how projects are going, analyzing the more minor details is essential. This becomes a challenge when there are dozens or hundreds of projects to go through. To solve this problem, simple reporting tools are utilized.
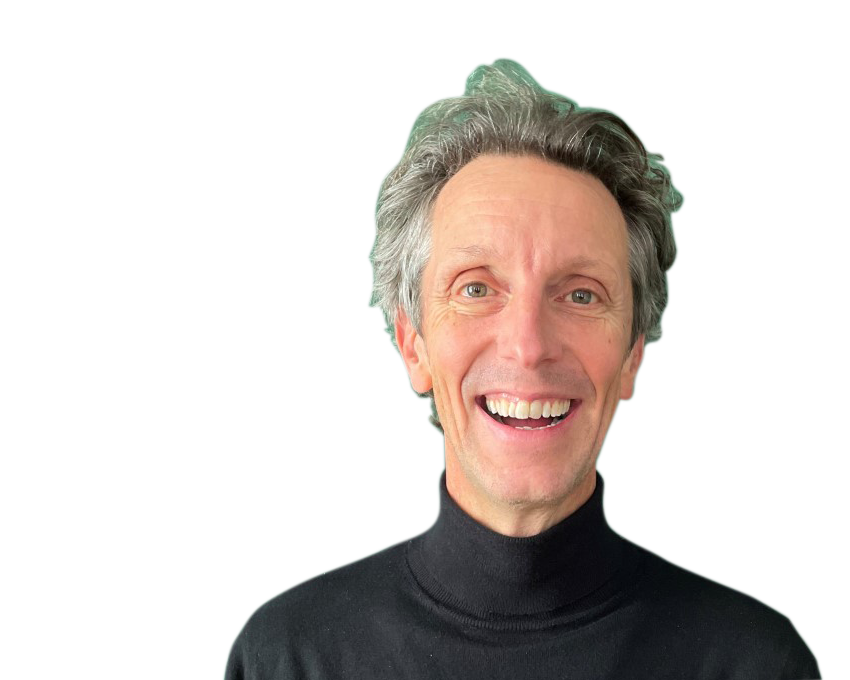
Mark van der Pas | CEO of Uffective
“My specialization is in Portfolio Management. Together with my team, we build and implement portfolio management solutions and help organizations to align their investments to their strategy, improve the financial impact of their IT portfolio, speed up time-to-market and simplify processes e.g., by reducing double work and creating one single version of the truth.”
A popular way to report the success of a project is with the traffic light system
This uses red, yellow, and green to analyze how a project functions, but when you reduce complex projects down to such simple and manually selected options, you can lose track of how well your project is functioning.
A significant issue is that project managers and product owners may also be reluctant to flag problems to senior management, which will attract attention.
So how does all of this relate to watermelons?
A watermelon project is a project that looks green and good to go on the outside but is red and failing on the inside. In other words, a watermelon project appears to be successful and well-managed, but in reality, it is not. They can also be projects on time and budget but no longer fulfill the initial business goals for which they were set up.
How can we flag that a project has the potential to become a watermelon project?
We use machine learning to help predict at an early stage whether a project will become a watermelon project. To do this, we don’t look at whether the project manager has managed a failed project before; we look at the previous success of other projects. By analyzing this data, we can see likely the project is to fail, however it may seem on the outside.
Using machine learning, we can evaluate how long it takes for a project that has a red signal to resolve its issues. Equally, if there are no red signals, this should be flagged too for closer inspection.
By using machine learning, we can overcome the problems with the traffic light system
The hugely beneficial thing about machine learning is that it can give you the assistance you need to make better decisions. It isn’t taking over responsibility or making choices for you, but it can give you vital information and help to eliminate human error. By using machine learning, Uffective can give you more transparency over what projects are succeeding and what aren’t and help you to overcome the problems inherent in the typical traffic light system.